Understanding the Significance of Medical Image Annotation in Modern Healthcare
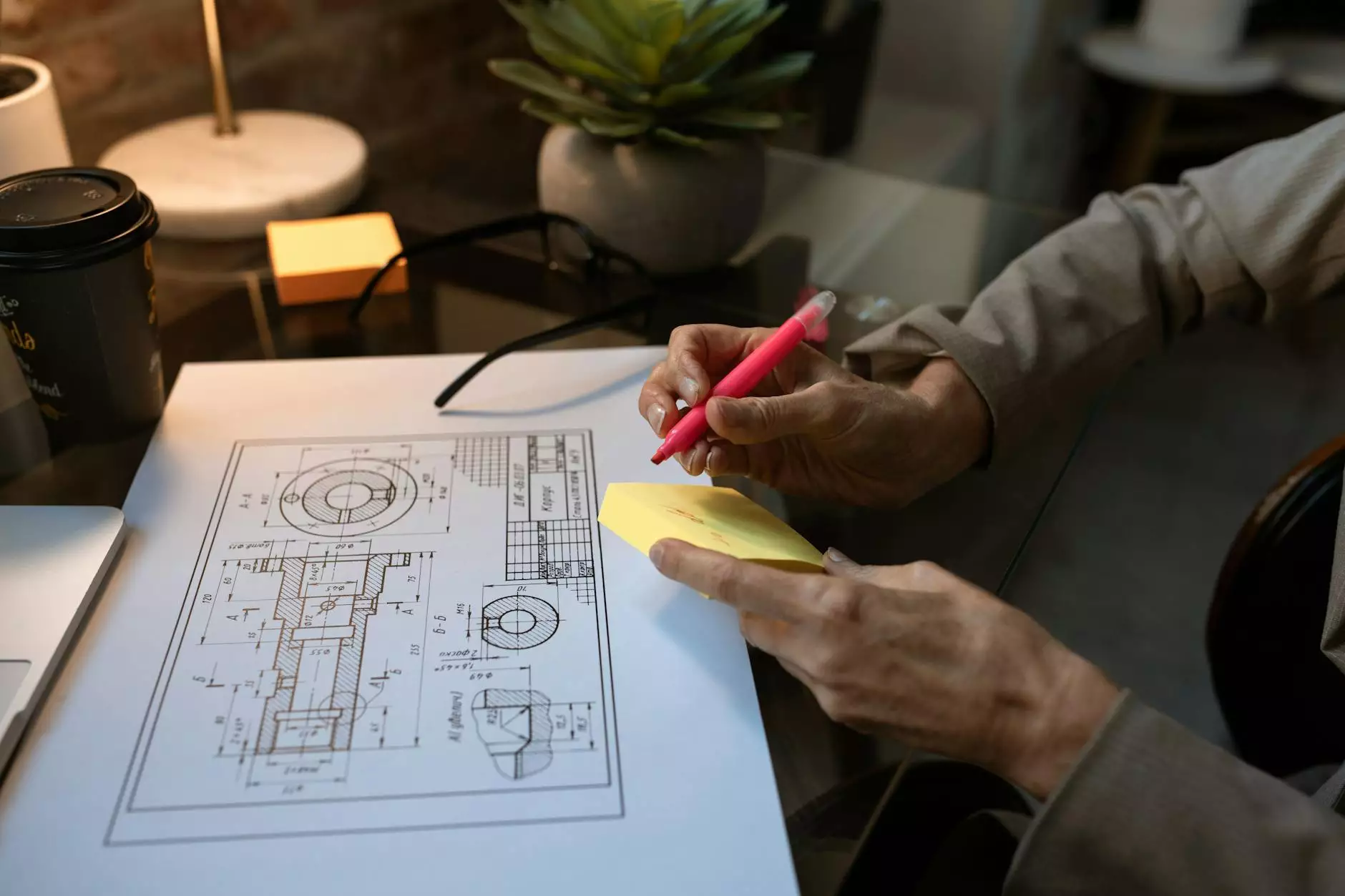
Medical image annotation is a crucial process in healthcare that involves labeling and organizing medical images to facilitate better understanding and usage in clinical practices. This process enhances the analysis of complex imaging data, allowing for more accurate diagnoses, research, and treatment planning.
The Importance of Medical Imaging
Technological advances in medical imaging have transformed how healthcare professionals diagnose, treat, and monitor patients. These imaging modalities include:
- X-rays - commonly used to view bone fractures and infections.
- CT Scans - provide detailed cross-sectional images of organs and tissues.
- MRI - utilizes magnetic fields to produce detailed images of soft tissues.
- Ultrasound - uses sound waves to create images of organs and blood flow.
What is Medical Image Annotation?
Medical image annotation refers to the process of labeling medical images to assist machine learning algorithms and healthcare professionals in understanding and interpreting the data accurately. This practice is fundamental in training artificial intelligence models that can automate and enhance diagnostic processes.
The Process of Image Annotation
The medical image annotation workflow typically involves several key steps:
- Image Acquisition - The process begins with capturing medical images using various imaging techniques.
- Pre-Processing - Images are prepared for annotation through techniques such as normalization and noise reduction.
- Annotation - Medical experts label images with relevant structures or abnormalities.
- Quality Assurance - Ensuring the accuracy and consistency of annotations through multiple reviews.
- Integration - The annotated images are integrated into databases for analysis and model training.
Applications of Medical Image Annotation
The applications of medical image annotation are vast and continue to expand as technology progresses:
1. Enhanced Diagnosis
Accurate annotations help radiologists and clinicians identify issues such as tumors, fractures, or other anomalies more effectively. This serves to improve diagnostic accuracy and patient outcomes.
2. Artificial Intelligence and Machine Learning
Annotated medical images are essential for training AI algorithms. Machine learning models, which require vast amounts of labeled data, benefit immensely from accurately annotated images, resulting in systems capable of assisting in image interpretation, prioritizations, and even autonomously identifying conditions.
3. Research and Development
In the research domain, medical image annotation is used to compile large datasets for studies examining disease patterns, treatment efficacy, and more. This data is crucial for the development of new diagnostic tools and therapeutic strategies.
4. Education and Training
Medical image annotation plays a significant role in the education of medical professionals. By providing annotated examples, learners gain insights into interpreting and analyzing medical imagery, essential for their professional competence.
Benefits of Medical Image Annotation
The benefits of implementing medical image annotation in healthcare are numerous:
- Improved Accuracy: Labeling images reduces the chances of diagnostic errors.
- Efficiency: Facilitates quicker reviews and decision-making in clinical settings.
- Facilitated Collaboration: Enhances communication between various healthcare specialists through clear references.
- Advancement of AI: Contributes to the growing capabilities of machine learning applications in healthcare.
- Higher Research Quality: Enriches datasets contributing to more reliable research findings.
Challenges in Medical Image Annotation
While the practice of medical image annotation boasts numerous benefits, several challenges remain:
- Time-Consuming: Manual annotation can be a lengthy process requiring significant expertise.
- Subjectivity: Different experts may interpret images differently, leading to inconsistencies.
- Data Privacy: Ensuring patient confidentiality while annotating images is crucial.
- High Costs: The technological and workforce investment can be substantial.
The Future of Medical Image Annotation
As technology continues to advance, the future of medical image annotation appears promising:
A. Automation
With the development of more sophisticated AI tools, the annotation process is expected to become more automated. This not only allows more images to be labeled in a shorter time frame but also reduces human error.
B. Enhanced Algorithms
Machine learning algorithms will continue to evolve, allowing for better predictive analytics in healthcare, ultimately leading to improved patient outcomes.
C. Interdisciplinary Collaboration
The combination of expertise in medical imaging, computer science, and data analytics will foster innovative solutions and applications in clinical settings.
Conclusion: The Transformational Role of Medical Image Annotation
In summary, medical image annotation serves as a foundational component in the evolution of healthcare. By improving diagnostic accuracy, facilitating AI development, and enhancing research, this practice is set to revolutionize how medical professionals operate. Investing in the refinement and innovation of medical image annotation techniques will pave the way for a brighter, more efficient future in healthcare.